Air Quality Impacts of New York State Cap-Trade-and-Invest Design
This issue brief, produced in partnership with the New York City Environmental Justice Alliance, conducts a robust air quality analysis of several different cap-trade-and-invest policy designs in New York.
1. Introduction
The New York Department of Environmental Conservation (DEC), the New York State Energy Research and Development Authority (NYSERDA), and the New York Governor are currently finalizing draft regulations that will determine how an economy-wide emissions cap-and-trade system (also referred to as “NYCI” by the State) will work in New York State. The regulations will influence how much and how equitably greenhouse gas (GHG) and copollutant emissions are reduced. Advocates, such as New York City Environmental Justice Alliance and New York Renews, referring to the program as “cap-trade-and-invest,” have been calling for guardrails in the program to make sure the State complies with the Climate Leadership and Community Protection Act (or the “Climate Act”), and that disadvantaged communities (DACs) do not experience more air pollution or slower, lower rates of air quality improvement compared to non-DACs. Advocacy groups have also been calling for regulations that will bolster the amount of program revenue for climate investment and action.
Our research to date has analyzed different cap-trade-and-invest policy designs and their corresponding GHG emissions, copollutant emissions, and household cost impacts. Previous work conducted by Resources for the Future (RFF) and New York City Environmental Justice Alliance (NYC-EJA), Krupnick et al. (2024) and Robertson et al. (2024), found that the cap-trade-and-invest program can reduce GHG and copollutant emissions. The research presented in this issue brief shows that guardrails, such as restricted trading, facility-specific caps, and obligating the electricity sector improve air quality for New Yorkers, especially those in DACs. Earlier reports offer more detail on overall emissions changes across the state and generator-level emissions changes in the power sector. Separate air quality modeling from the emissions modeling was needed because the relationship between direct emissions and local air quality is highly affected by complex chemical processes and atmospheric conditions.
In this report, we leverage the emissions findings reported in Krupnick et al. (2024) and Robertson et al. (2024) to conduct a robust air quality analysis using a state-of-the-art atmospheric model initially used in Krupnick et al. (2023). Emissions changes in sulfur dioxide, nitrous oxides, and direct PM2.5 are used to estimate local PM2.5 concentrations at a 4km2 grid resolution. We focus on PM2.5 concentrations because this pollutant is tied directly to health and wellness outcomes, and small changes in concentrations can lead to meaningful impacts on mortality and chronic disease (e.g., asthma) rates (Di et al. 2017; Krewski et al. 2009; Lepuele et al. 2012).
This analysis compares tract-level air quality estimates for four policy cases:
- The Business as Usual (BAU) case, which includes the NY Clean Energy Standard, the Regional Greenhouse Gas Initiative (RGGI), Inflation Reduction Act (IRA) policies, renewable generation mandates, zero emissions vehicle mandates, and other existing policies. In this case, there is no cap-trade-and-invest program.
- The Electricity not Obligated Case (ENOC), where cap-trade-and-invest is implemented in New York State, but the electricity sector is not covered. Power sector facilities in the state are still required to purchase allowances from the RGGI market.
- The Full Trading Case (FTC), where cap-trade-and-invest is implemented, electricity is covered under cap-trade-and-invest, and there is full trading across sectors.
- The Restricted Trading Case (RTC), where cap-trade-and-invest is implemented, electricity is covered under cap-trade-and-invest, and there are sector-specific caps and facility-specific caps on power generators that constrain trading.
Our air quality analysis reveals:
- Each modeled cap-trade-and-invest policy design delivers air quality benefits across a variety of community types in New York State. The greatest air quality improvements for disadvantaged communities and all other communities are delivered by the RTC, and the smallest benefits are delivered by the ENOC.
- The greatest average air quality improvements in all cases are found in disadvantaged communities, particularly those with high historic air pollution, high vulnerability scores, or high environmental burden scores on the state’s disadvantaged community index.
- The greatest air quality improvements in all cases are in New York City.
- In each policy case, a small fraction of tracts experience smaller air quality improvements than those expected in the BAU. The ENOC has the greatest number of tracts with these smaller improvements, while the FTC has the least.
2. Methodology
The policy cases analyzed in this work were developed by a team of researchers at RFF and NYC-EJA. The policy cases are designed to assess the overall emissions and air quality impact of a cap-trade-and-invest program and the impact of specific program guardrails under consideration to improve outcomes for disadvantaged communities. NYC-EJA offered their expertise on the New York policy landscape and the specific policy questions most relevant to disadvantaged communities as the program design continues to take shape.
The PM2.5 concentrations in this analysis were produced using an air quality model that leverages emissions estimates from the power, transportation, and residential buildings models presented in Krupnick et al. (2024) and Robertson et al. (2024). Details on the energy models, which produce estimates of emissions changes in the power, transportation and residential buildings sectors, can be found in the appendices of Krupnick et al. (2024).
Two air quality models are used to estimate PM2.5 concentrations at a grid resolution of 4km2 across New York State for 2030 under the four policy scenarios. The first is the Weather Research and Forecasting model coupled to chemistry (WRF-Chem v4.3). WRF-Chem is a state-of-science online-coupled 3-D air quality model containing comprehensive representations of atmospheric transport, physics, and chemistry to simulate PM2.5 concentrations. It was applied at a grid resolution of 36km2. The second model used is a computationally efficient statistical model utilizing the 36km2 simulation results from the 3-D air quality model to predict PM2.5 concentrations at a grid resolution of 4km2. Appendix C in Krupnick et al. (2023) offers greater detail on the air quality modeling methodology used in this work.
A spatial mapping and interpolation method is used to translate 4km2 PM2.5 concentrations into tract-level concentration estimates. The annual average PM2.5 concentration is generally used to compare air quality outcomes across policy cases in our analysis, with the exception of Figure 4 and Table 2, which report the eighth-highest daily PM2.5 concentration for different communities (reflecting the National Ambient Air Quality daily maximum standard—the 98th percentile daily value over three years).
3. Results
Figure 1 reviews the economy-wide emissions results from Krupnick et al. (2024) and Robertson et al. (2024) that drive the air quality results. Those previous analyses found that cap-trade-and-invest without electricity sector obligation (the ENOC) could lead to economy-wide emissions reductions, but emissions increase in the power sector driven by load growth. Obligating the electricity sector and allowing free allowance trading across all sectors (the FTC) leads to emissions reductions in each modeled sector but does not guarantee emissions reductions for all sectors and power sector facilities. Adding facility-specific caps and sector-specific caps (the RTC) leads to some redistributions of emissions across sectors and requires each power sector facility and each sector to meet a minimum emissions reduction trajectory.
The emissions plots in Figure 1 show that GHG and copollutant differences between the policy cases are small, the exception being that there are relatively significant differences in electricity sector emissions based on whether the sector is obligated (the ENOC compared to other policy cases). Slight differences in the transportation sector results are evidence of the role of the zero emissions vehicle mandates included in the BAU and the other policy cases. Emissions pricing and electrification subsidies do not lead to significantly greater emissions reductions in LDV or MHDV sectors. The GHG emissions results are discussed and compared more completely in Krupnick et al. (2024) and Robertson et al. (2024). This report focuses on how the copollutant emissions associated with each policy case change local air quality in New York State.
Figure 1. Sectoral Emissions by Policy Case
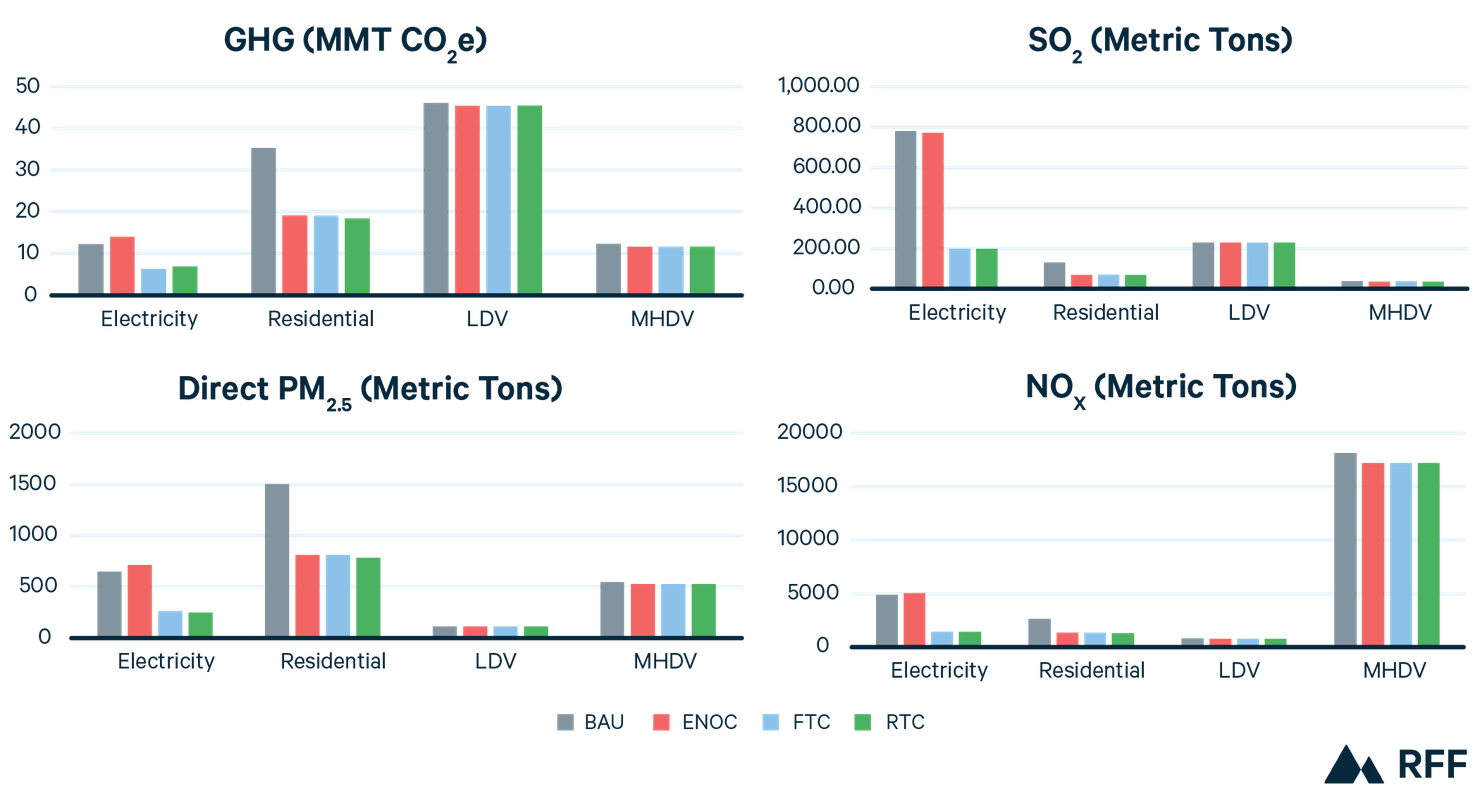
The maps in Figure 2 show PM2.5 concentration changes from BAU across each modeled design of cap-trade-and-invest. Yellow tracts represent negligible air quality changes, while dark blue represents the greatest air quality improvements. Tracts that are orange experience lower air quality than the BAU. These “deteriorations”, where they exist, are small relative to the average improvements over the BAU. In all policy cases, improvements are most dramatic in the New York City region, with other urban centers experiencing noticeable air quality improvements as well. Note that changes (improvements and deteriorations) are relative to the 2030 BAU case, not current air quality levels. The 2030 BAU includes several existing decarbonization policies in New York State that are anticipated to improve air quality (Krupnick et al. 2023).
The ENOC has the greatest estimated number of tracts with lower air quality than the BAU, with 14 percent of tracts experiencing 0.01 μg/m3 higher PM2.5 concentrations than the BAU. The RTC has many fewer estimated tracts with air quality deteriorations from BAU, with 6 percent of tracts experiencing 0.01 μg/m3 higher PM2.5 concentrations than the BAU mostly in the west of the state. The FTC has the fewest estimated tracts with air quality deteriorations, with just 2 percent of tracts experiencing 0.01 μg/m3 higher PM2.5 concentrations than the BAU. The RTC shows the greatest air quality improvements over the BAU, with a maximum PM2.5 concentration reduction of 0.29 μg/m3, and 33 percent of tracts experiencing estimated concentration reductions greater than 0.2 μg/m3. In contrast, the FTC has 24 percent of tracts with an estimated PM2.5 reduction greater than 0.2 μg/m3, and the ENOC only has 8 percent of tracts in that category. More detail can be seen in the distribution plots in Figure 3.
Figure 2. Air Quality Changes from BAU to Each Policy Case, 2030
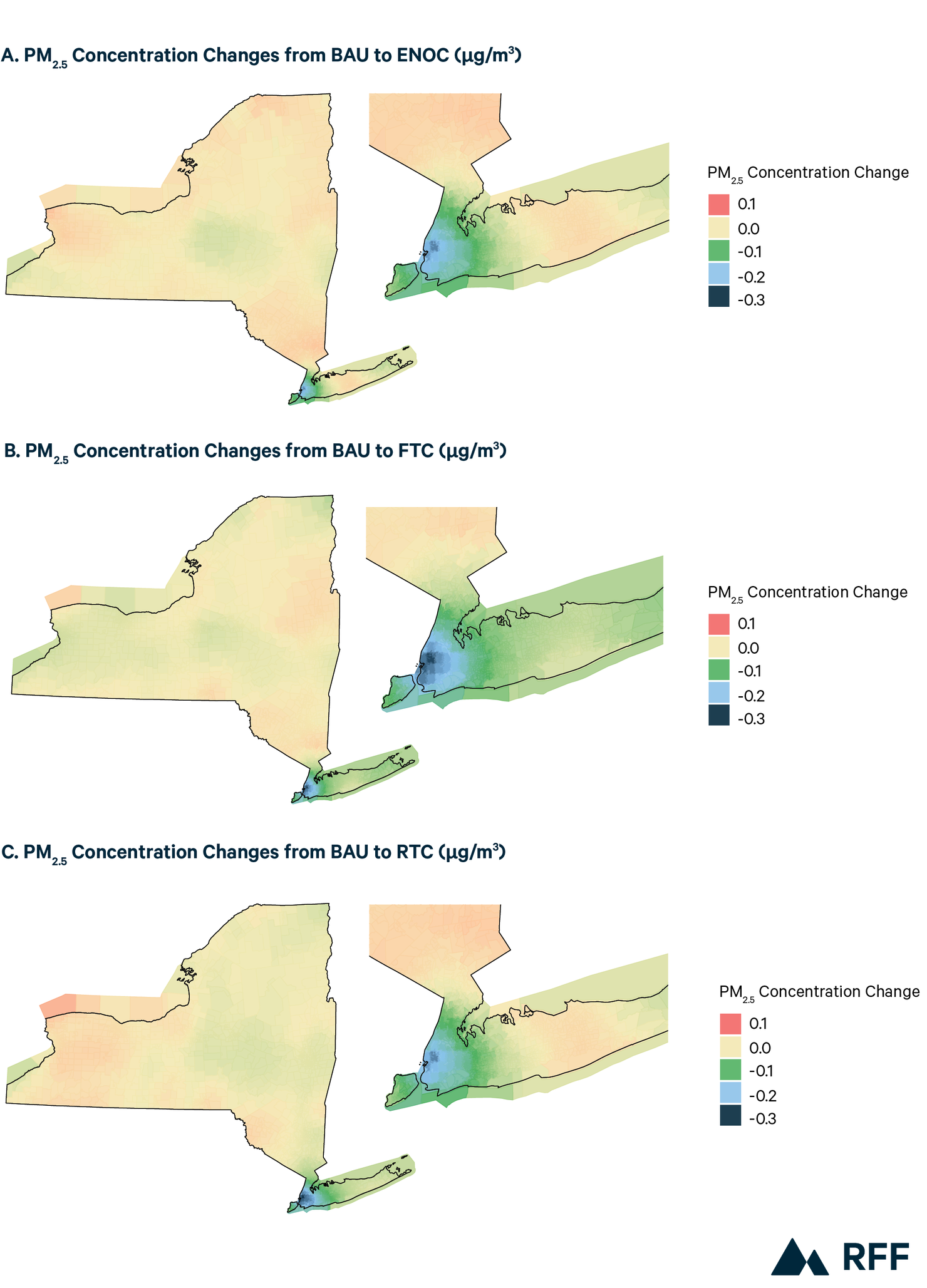
Figure 3 illustrates the distribution of air quality changes in New York State for all tracts (left) and for DAC tracts only (right ). The plots show higher “density” when a relatively higher number of census tracts experience the PM2.5 concentration change represented on the x-axis. A horizontal line would represent a perfectly even distribution of air quality changes. The plots reveal a roughly bi-modal distribution, with a high concentration of tracts experiencing little to no air quality improvements (the peak at 0 μg/m3) and another cluster of tracts near the maximum air quality improvements (the peak around -0.2 μg/m3). This pattern holds when only DAC tracts are analyzed (in the right panel). The shape of the distributions is consistent across policy cases, but the trends observed in the maps in Figure 2 can be seen clearly here as well: the RTC has the most tracts experiencing high air quality improvements relative to the BAU, while the ENOC has the highest number of tracts experiencing no improvement or lower air quality improvements than the BAU. The RTC has slightly more tracts than the FTC that experience lower air quality improvements than the BAU, but the community-level results in Table 1 indicate that the RTC still has greater average improvements. Regional concentrations of lower air quality improvement could be justification for greater investments or use of revenue to spur electrification and further emissions reductions. We do not have evidence of net emissions increases in New York between the BAU and RTC, so lagging air quality improvements are likely the result of non-linear relationships between copollutant emissions (including those out of state) and PM2.5 concentrations.
Figure 3. Quality Changes from BAU, by Policy Case
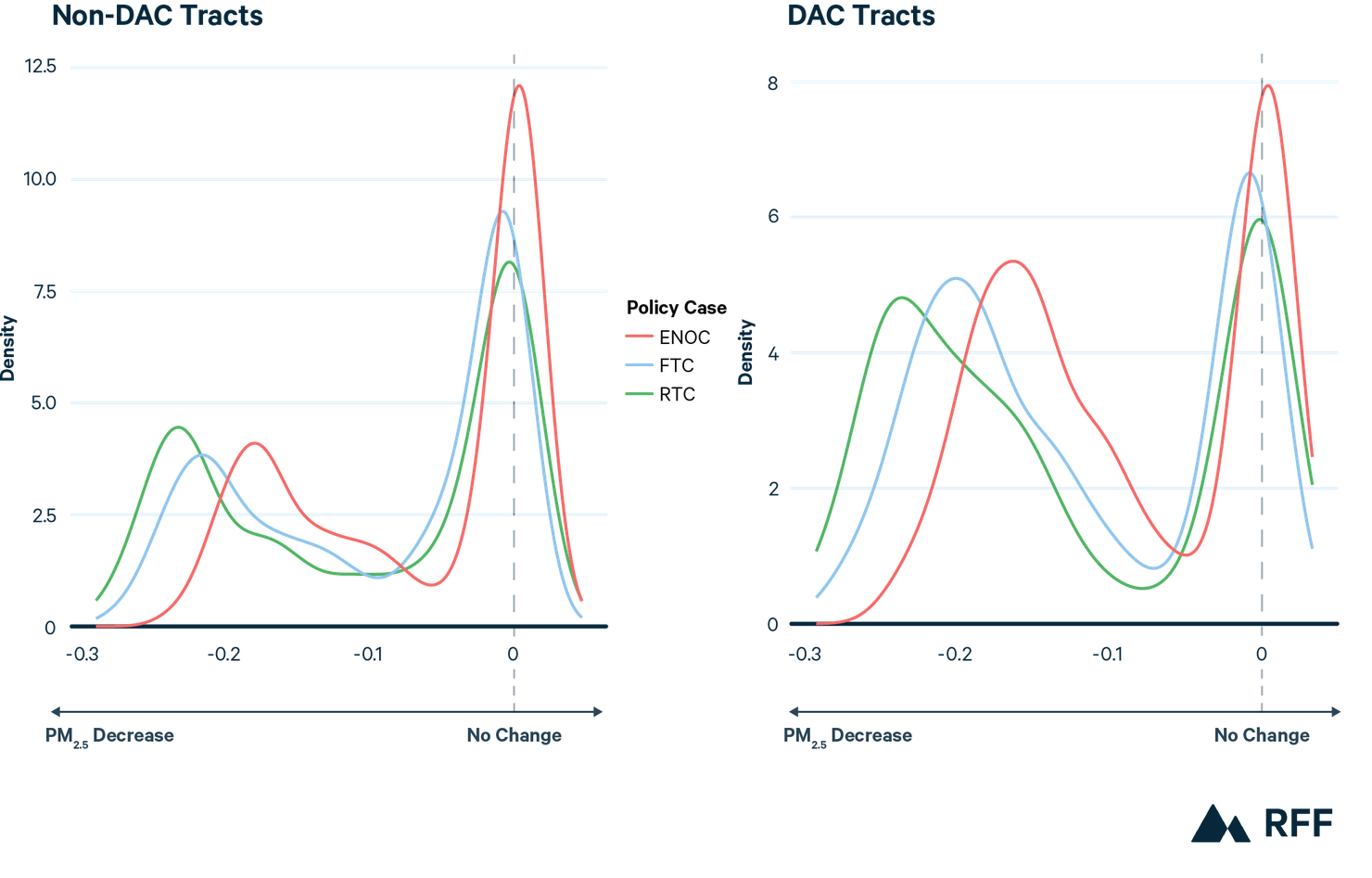
In Krupnick et al. (2022), we found that different implementations of the Climate Act could lead to meaningful differences in air quality across community types. While the disparity in improvements between DACs and non-DACs is of interest, we also found that specific sub-categories of communities disproportionately benefited from a more ambitious policy implementation. Using the state’s disadvantaged community index, we also analyze the average air quality changes in communities that scored in the 90th percentile on environmental burden, socioeconomic vulnerability, elderly population, and historic PM2.5 levels.
Table 1 reports the population weighted average PM2.5 concentration change from the BAU to each cap-trade-and-invest policy case. For each community type, the greatest reduction in PM2.5 concentrations is observed in the RTC, and the smallest reduction is observed in the ENOC. While different community types experience different concentration changes than the average, the difference between policy cases is relatively uniform. In other words, the air quality improvements in the FTC and the RTC, relative to the ENOC, are roughly the same across subcategories of communities.
Table 1. Change in Annual Average* PM2.5 Concentration from BAU 2030, by Policy Case (μg/m3)
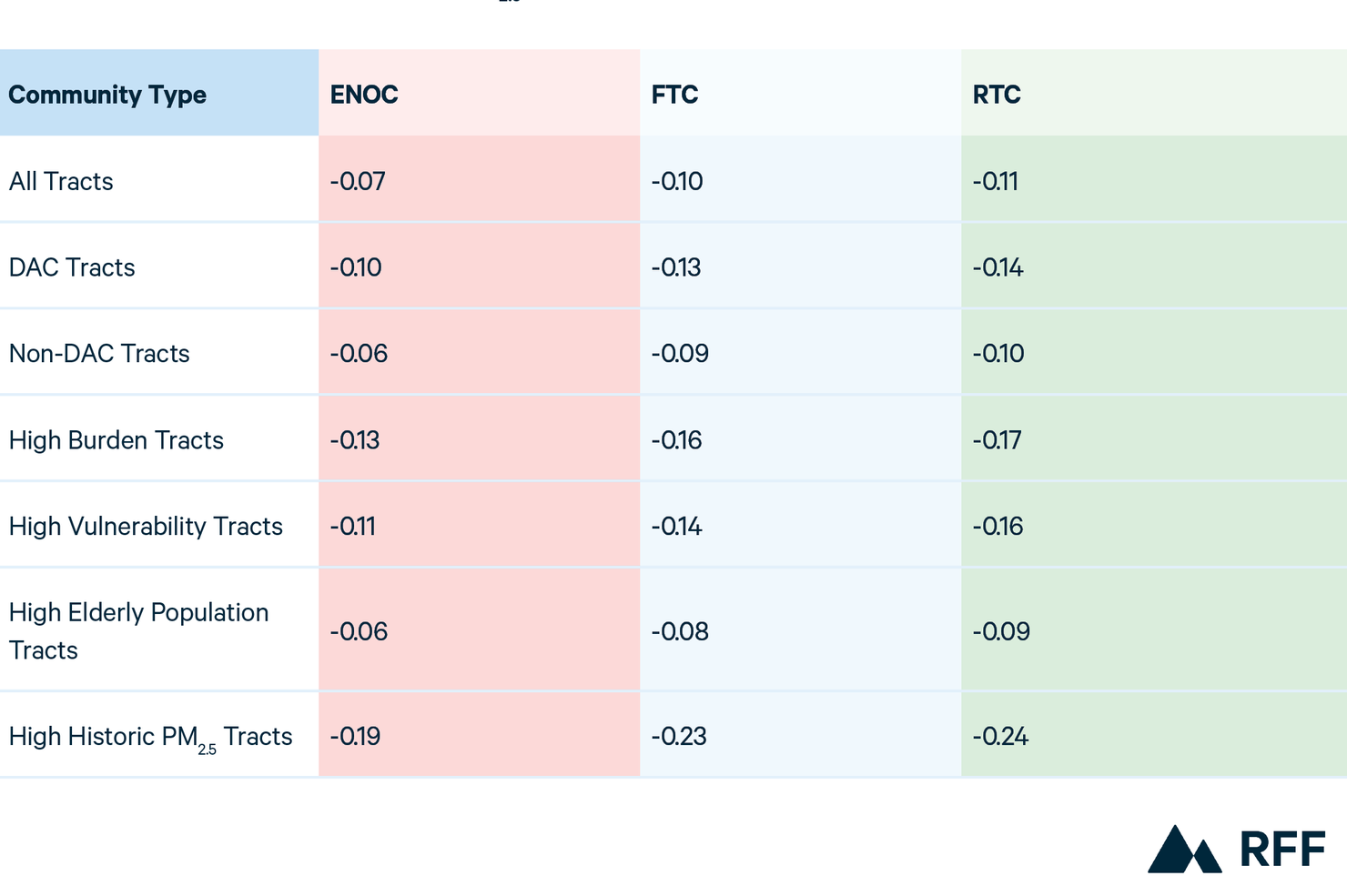
*averages weighted by tract population.
Note: More negative values indicate greater PM2.5 concentration reductions relative to BAU.
We also measured changes in “daily maximum” PM2.5 concentrations, which focus on days with the worst air quality rather than the annual average. Because of how “design values” for non-attainment designations are defined, daily maximums are calculated using the 98th percentile concentration over three years of observations. Because we only model air quality in one year (2030), we adjust the metric to select approximately the 98th percentile of the estimated 2030 daily concentrations (2% of 365 days rounded up). Daily maximums are, by definition, much larger and more variable than annual average changes (presented in Table 1) but can still be important in assessing overall air quality impacts.
Figure 4 shows the density distribution of daily maximum PM2.5 concentration changes by census tract. The daily maximum values for tracts are much more variable than the annual averages presented in Figure 3. While the ENOC still reveals the most tracts with lower air quality than the BAU, when comparing the FTC to the RTC, there is a relatively large spike in daily maximum air quality improvements in the FTC. This is true for DAC and non-DAC communities and is distributed across the state. Since we are only modeling one year and can’t follow the standard practice of averaging across multiple years, this may be an outlier observation.
Figure 4. Quality Changes from BAU, by Policy Case
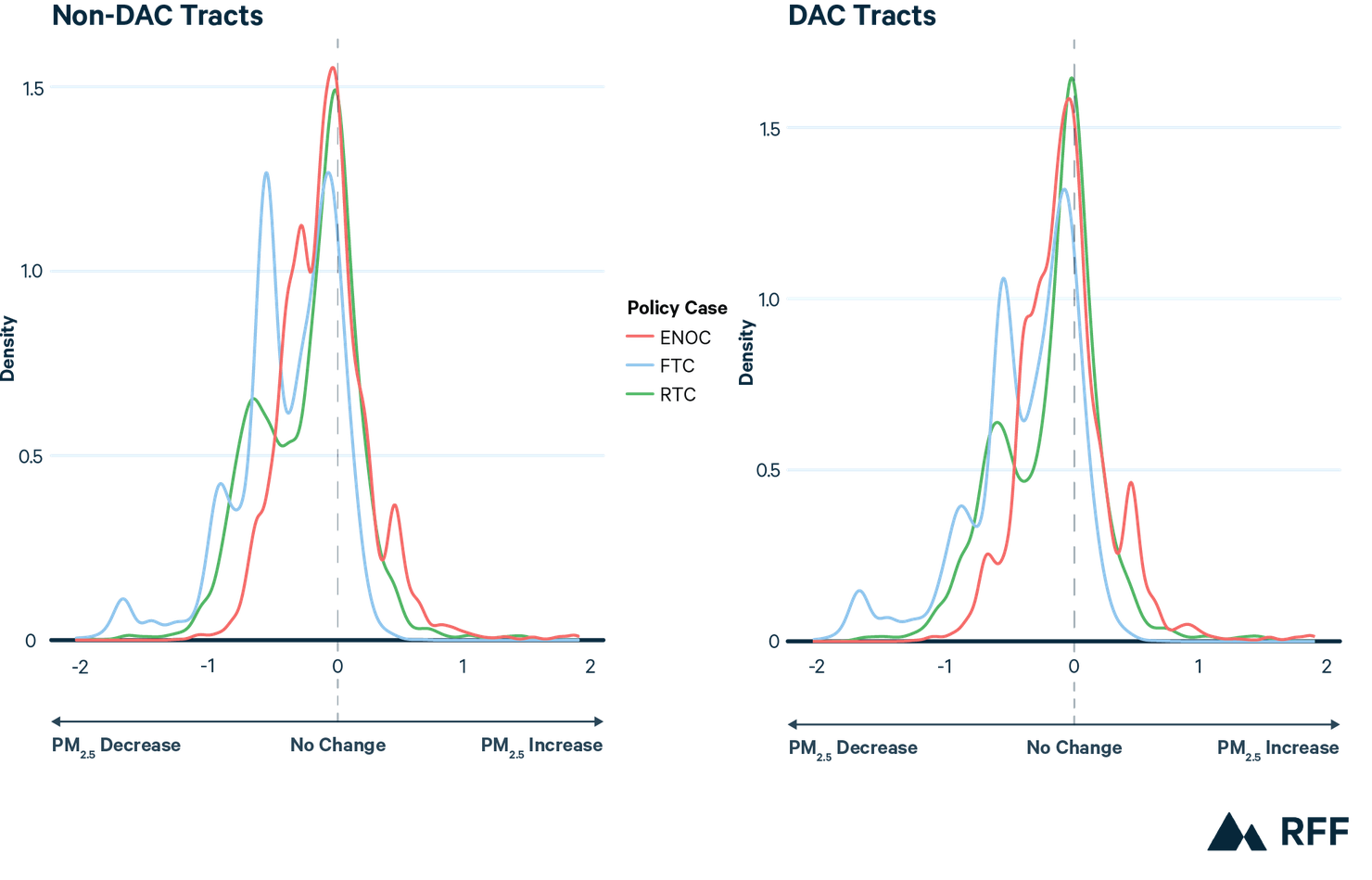
This pattern is corroborated by the community-type PM2.5 concentration averages shown in Table 2. Across all community types, the FTC delivers the greatest reductions in the daily maximum PM2.5 concentrations. The ENOC delivers the lowest reductions in the daily maximum PM2.5 concentrations. The magnitude of these differences may seem large compared to the comparisons presented in Table 1, but note that daily maximums are by definition much larger than annual averages. The national standard for daily maximum concentrations is more than double that for annual average concentrations.
Table 2. Change in High Daily* PM2.5 Concentration from BAU 2030, by Policy Case (μg/m3)
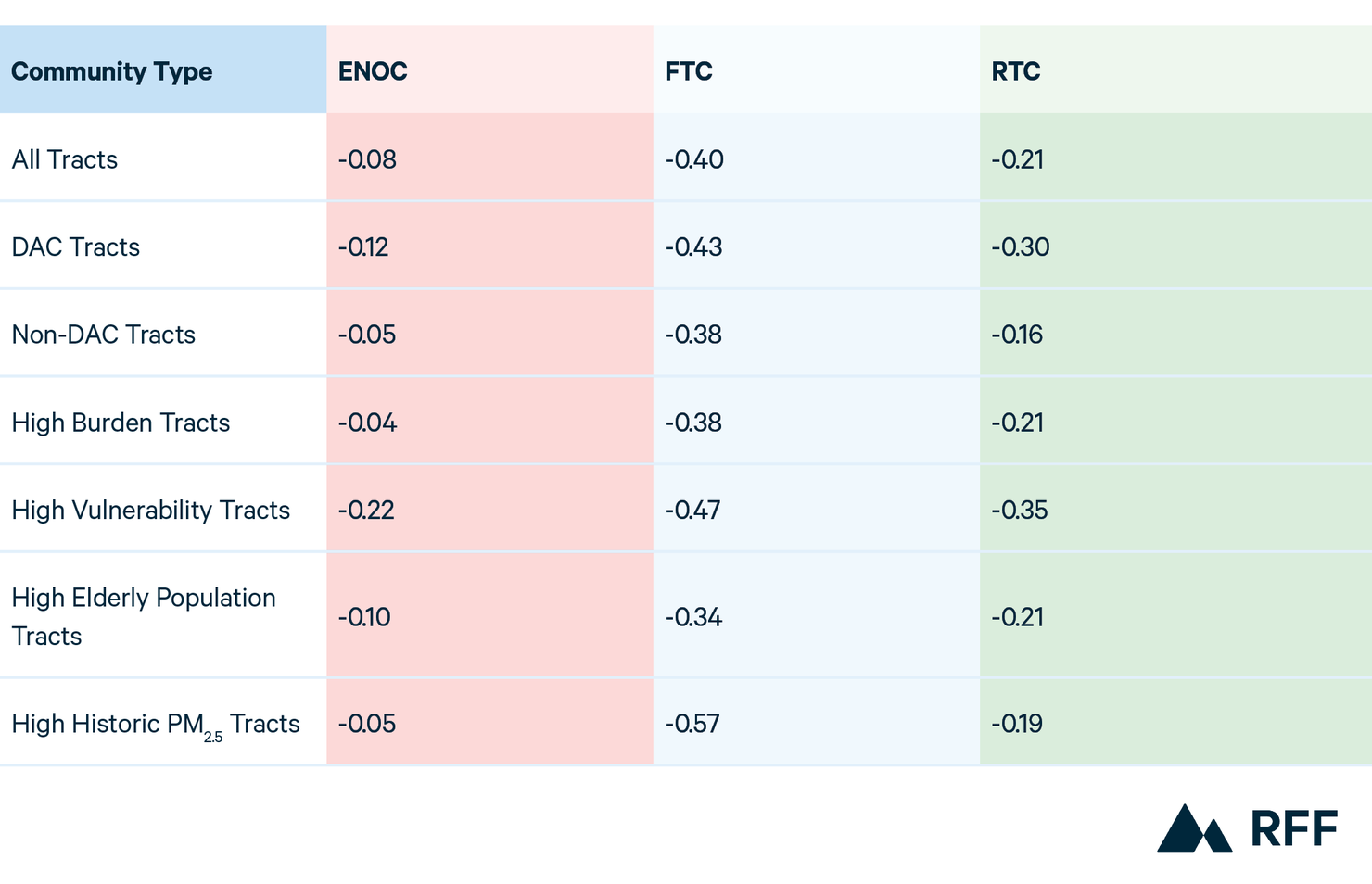
*Value represents weighted average of the eighth-highest concentration day in 2030 for each tract
Note: More negative values indicate greater PM2.5 concentration reductions relative to BAU.
The NYSERDA and DEC preliminary analysis of the cap-trade-and-invest program offers some context for how these air quality improvements may relate to health outcomes (NYSERDA & DEC 2024). In the data annex for the state’s analysis, state-wide annual average PM2.5 improvements are connected to changes in mortality risk, nonfatal heart attacks, hospitalizations, acute bronchitis, respiratory systems, emergency room asthma visits, asthma exacerbation cases, restricted workdays, and lost workdays. For a statewide PM2.5 concentration decrease of 0.11 μg/m3, like that observed in the RTC compared to the BAU (see Table 1), the state estimates between $1.4 billion and $3.0 billion in health benefits. Additionally, in Krupnick et al. (2023), our team conducted an analysis to identify the potential health benefits of air quality improvements associated with Climate Act implementation. We found that for an average concentration reduction of 0.18 μg/m3, with higher reductions in population-dense areas like New York City, the estimated mortality reduction benefits were around $2 billion in 2030. The distribution of air quality improvements in Table 1 indicates that disadvantaged communities could realize a significant share of these benefits. More detailed research on the health impacts, that draws on specific knowledge about New Yorkers’ health and historic responses to pollution would be extremely valuable in assessing the ultimate impact of these policies.
4. Assumptions and Caveats
The details and assumptions made in our modeling may affect the interpretation of results. Below are some relevant details on our modeling.
- RGGI. New York is assumed to stay in RGGI in all policy cases. In the cases where electricity is obligated, generators are credited for the RGGI price under cap-trade-and-invest. RGGI is modeled as a price rather than a cap, because it is assumed that RGGI prices will be at emissions containment reserve levels (the price floor) when Pennsylvania is included. Since completing our modeling, RGGI has begun its program review, which may lead to higher prices. Higher RGGI prices could affect emissions across all policy cases, but particularly in the BAU and the ENOC by reducing emissions in the baseline and limiting the additional emissions reductions associated with introducing cap-trade-and-invest.
- New Generation Builds. The state’s preliminary scenario analyses (NYERDA & DEC 2024) assume constraints on new generation to reflect interconnection queues and permitting delays. They lift these constraints in 2031. This assumption allows them to observe differences between economic responses in the early years compared to the later years of the program. We only model 2030 and do so without these constraints (thus making a comparison between our 2030 results and their 2031 results more appropriate). Because we do not model prior year constraints, our model allows for greater expansion of renewables. Capacity additions for each policy case can be found in Robertson et al. (2024)
- Sectoral Coverage. We assume no emissions changes from the historic baseline for the sectors we do not model. This limits the air quality improvements estimated to those associated with emissions changes in our modeled sectors. Non-linearities in the relationship between emissions and air quality mean that emissions changes in other sectors could affect the air quality changes in unexpected ways.
5. Conclusion
Our analysis describes the local air quality effects of various cap-trade-and-invest design cases, including those with guardrails prioritized by environmental justice stakeholders in New York State. On average, the RTC delivers the greatest annual average PM2.5 reductions across all community types in New York State and leads to the greatest number of tracts experiencing a concentration reduction greater than 0.2 μg/m3. The FTC delivers the greatest improvements to daily maximum concentrations and minimizes the number of tracts that experience PM2.5 concentration increases of more than .01 μg/m3. The ENOC is the worst performing policy case on air quality metrics predicted by our model.
Of the guardrails tested, obligating the electricity sector has the largest impact on air quality. Without electricity obligation, roughly 14% of New York census tracts experience smaller air quality improvements than the BAU. As policymakers consider the design of cap-trade-and-invest, it is important to evaluate how even small changes in program design may impact the distribution of emissions and, ultimately, community air quality.
The CLCPA requires regulators to ensure investments and benefits are delivered to disadvantaged communities, and that the programs implemented to achieve climate goals in the state do not cause pollution and harm. Our analysis reveals that program design has an impact on the magnitude of air quality benefits and the prevention of instances of lower air quality than in the BAU.
Authors
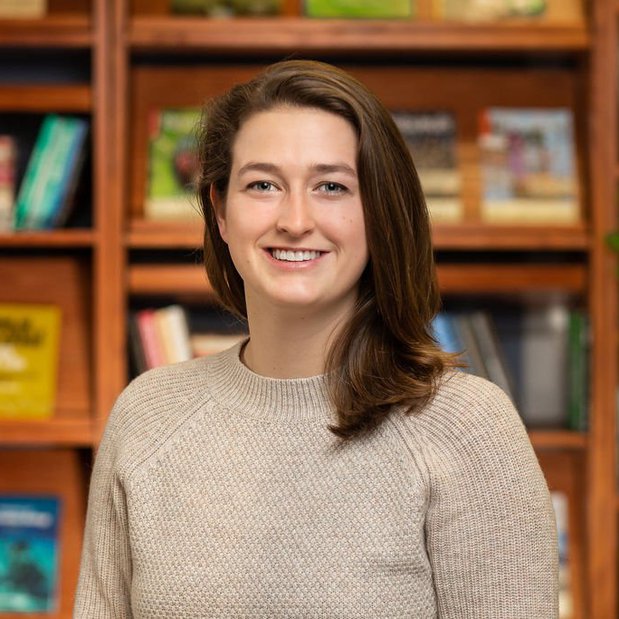
Molly Robertson
Associate Fellow
Molly Robertson is an associate fellow at Resources for the Future.
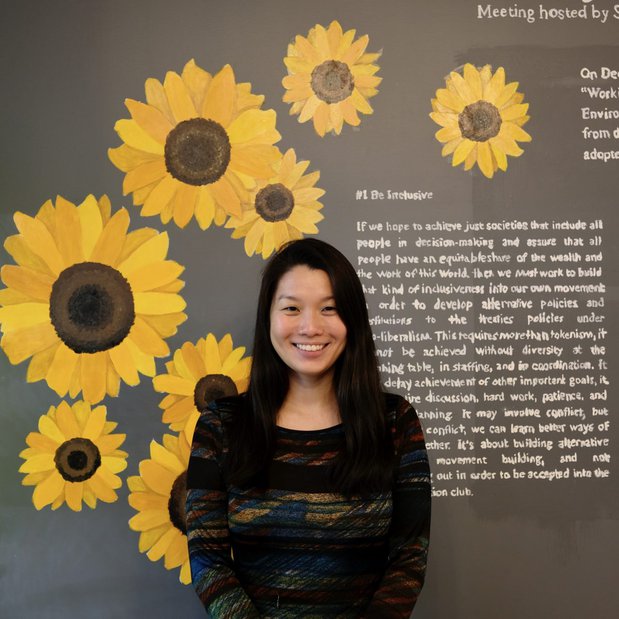
Eunice Ko
New York City Environmental Justice Alliance
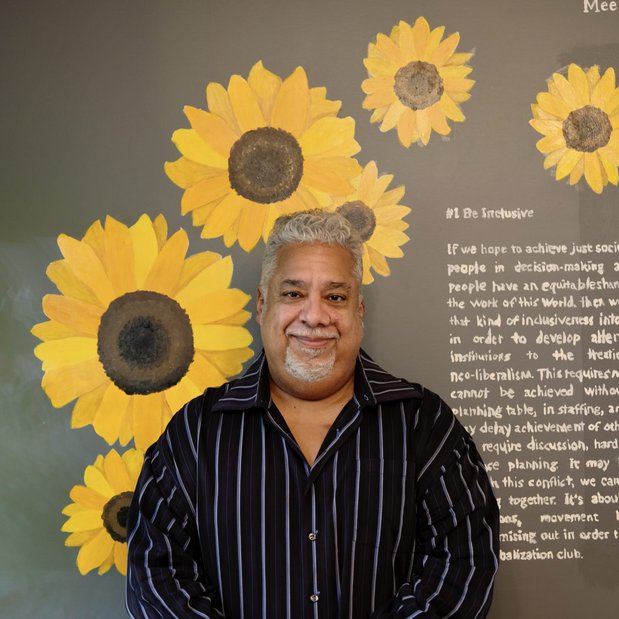
Eddie Bautista
New York City Environmental Justice Alliance