Weather Variability Explorer
Explore a database of decision-relevant weather variability indicators developed by Resources for the Future.
Introduction
Climate change is altering weather patterns around the globe. Consequentially, average values of temperature, rainfall, and other weather conditions are shifting over time. These impacts are not the same everywhere; some communities are experiencing wetter conditions while others are suffering through greater droughts. Beyond changes in average weather conditions, climate change is increasing weather variability and the frequency and severity of extreme events such as heavy rainfall, heat waves, and wildfires.
While weather variability has been a lived experience for many communities for a long time, climate change exacerbates its scale and impacts. To date, there have been challenges in properly assessing the risks posed by weather variability and developing needed adaptation and resilience strategies. Individuals, community leaders, industries, and policymakers need information at their disposal to better understand and address weather variability and extremes.
The Weather Variability Explorer (WeaVE) is a database of decision-relevant weather variability indicators developed by Resources for the Future (RFF). WeaVE highlights how precipitation, temperature, heat waves, and fire events have varied over space and time in the past four decades. The database also shows how weather variability and extremes are expected to evolve with climate change.
Data Tool
Exploring WeaVE
The map shows geographic patterns in weather variability and extremes across the United States. The timeline shows how each weather variability indicator has evolved in the recent past and can be expected to evolve with climate change in a given location.
The dropdown menus allow users to adjust the information displayed on the map. Users can view weather variability indicators for precipitation, temperature, heat waves, and fire events. Users can further select the indicator of interest, spatial scale (high-resolution grid, county, state, or national) and temporal resolution (annual or five-year aggregates). Users have the option to view historical measures (1981 to present) or projections under climate change (2020 to 2100). On the maps, users can select which date they would like to view. On the timelines, users can select their location of interest.
Users have the option to zoom into the map by double clicking. Hovering shows the location and indicator value.
Indicator definitions are below, along with data sources.
To better understand the data tool, let’s look at heat waves.
The map shows the number of heat waves per year across the United States. We can see that the average American experienced six heat waves in 2016 (by selecting “National” as the spatial scale), but many counties in the southeastern United States experienced upward of ten heat waves that year (by selecting “County” as the spatial scale). The timeline below the map shows that the number of heat waves has been increasing over time since 1980 (by selecting “Historical Observations” as the source). We can further see that climate change is expected to nearly double heat wave exposure by the end of the century (by selecting “Projections” as the source).
Through RFF Data Commons, a new partnership between Google and RFF, users can seamlessly explore relationships between weather variability and a wide range of other environmental and sociodemographic data. For example, users can view the association between extreme heat exposure and community-level resilience to natural disasters. These data show that heat waves tend to disproportionately affect communities that are less prepared and less equipped with the tools needed to mitigate the negative impacts of extreme heat.
About the Indicators
To understand the risks posed by weather variability, we must be able to measure the frequency and intensity of extreme weather conditions. From a physical science perspective, it is difficult to devise a comprehensive metric that encompasses all aspects of weather variability and extreme behavior in the climate. The research community, therefore, uses a range of indicators that describe variability in individual climate variables and summarize exposure to extreme events.
WeaVE provides two types of indicators that rely on different statistical measures (described below), both of which are used by the research community.
Empirical dispersion and shape statistics. WeaVE displays four variability metrics commonly used to measure the magnitude of variability, asymmetry of variability, and frequency of extreme deviations.
- Standard deviation. The standard deviation measures the amount of variation in a set of values, or the magnitude of volatility. A low standard deviation indicates that values tend to be close to the mean (low variability) while a high standard deviation indicates that values are spread over a wider range (high variability).
- Skewness. Skewness measures the asymmetry of a distribution. It indicates the propensity to experience positive or negative shocks.
- Kurtosis. Kurtosis measures the frequency of extreme deviations. It gives an indication of how likely a variable is to take very high or low values.
- Interannual range: The interannual range measures the spread between the minimum and maximum monthly temperature or precipitation in a given location.
Extreme event indicators. WeaVE displays a number of extreme event indicators that cover floods, droughts, heat waves, and fires.
- Standardized precipitation index (SPI). The SPI is a widely used index to characterize drought or abnormal wetness. It quantifies observed precipitation as a standardized departure from the historical distribution. On short timescales, the SPI is closely related to soil moisture. On longer timescales, it can be related to groundwater and reservoir storage.
- Extreme single-day precipitation events. This indicator measures the portion of total precipitation that occurred on days with extreme precipitation, where “extreme” is defined by the 90th percentile of precipitation that year. It describes what percentage of rainfall is arriving in short, intense bursts.
- Consecutive dry days. This indicator measures the maximum number of consecutive days with precipitation below 1 millimeter. Consecutive dry days have been shown to be a predictor of agricultural yields and ecosystem health.
- Heat wave count, length, and intensity. WeaVE reports three heat wave measures: the number, mean duration, and mean intensity of heat waves. In line with the US Environmental Protection Agency, we define a heat wave as a period of two or more consecutive days when the daily minimum temperature exceeds the 85th percentile of historical July and August temperatures for that location. Duration is measured as the mean length of heat waves, in days. Intensity is measured by how much observed temperatures exceed the local threshold, in degrees Celsius, during heat waves. For example, if a location has an 85th percentile of 30°C and the average daily minimum temperature during the heat wave is 32°C, the intensity would be 2°C.
- Fire events. WeaVE reports the spatial extent of fires in acres and in percent of the administrative unit (e.g., county, state) that burned. The database also includes estimates of PM2.5 concentrations attributable to wildfire smoke. We report average annual concentrations, measured in milligrams per cubic meters, and the number of days with unhealthy PM2.5 levels attributable to wildfire smoke. We define “unhealth days” as those with concentrations above 35 milligrams per cubic meter, a threshold above which PM2.5 is considered unsafe to human health and the environment and is in violation of the National Ambient Air Quality Standards (NAAQS) established by the Clean Air Act.
Data Sources
Weather variability indicators were computed by RFF researchers using the following datasets.
Precipitation, temperature, and heat waves: We use data on historical (1981-2022) precipitation, minimum temperature, and maximum temperature from the PRISM Climate Group, University of Oregon. Data are available at: https://prism.oregonstate.edu/historical/
Fire-burned area: Area burned statistics are computed using data from the USGS Monitoring Trends in Burn Severity project. Data are available at: https://www.mtbs.gov/
PM2.5 from wildfire smoke: Estimates of PM2.5 concentrations attributable to wildfire smoke come from Childs et al, “Daily local level estimates of ambient wildfire smoke PM2.5 for the contiguous US” Environmental Science & Technology, 2022. Data are available at: https://www.stanfordecholab.com/wildfire_smoke
Climate change projections: We use probability-weighted ensembles of US county-level precipitation, minimum temperature, and maximum temperature for the period 2020 to 2100 from Rasmussen et al, “Probability-weighted ensembles of US county-level climate projections for climate risk analysis” Journal of Applied Meteorology and Climatology, 2016.
Our projections are based on the HadGEM2 climate model Earth-System configuration under Representative Concentration Pathway (RCP) 4.5. RCP4.5 is a moderate emissions scenario in which emissions peak around 2040 and then decline. Data are available at: https://rucore.libraries.rutgers.edu/rutgers-lib/51702/#package
Authors
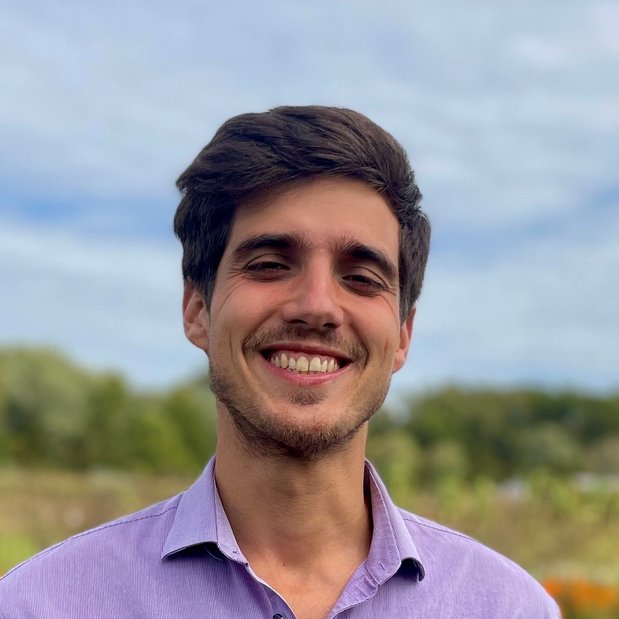
Billy Babis
Stockholm Environment Institute